Americans seeking medical care expect a certain level of privacy. Indeed, the need for patient privacy is a principle dating back to antiquity, and is codified in U.S. law, most notably the Privacy Rule of the 1996 Health Insurance Portability and Accountability Act (HIPAA), which establishes standards that work toward protecting patient health information.
But the world of information is rapidly changing, and in this environment, U.S. rules fall precariously short in protecting our medical data.
What many patients do not know is that, today, much of their health information is routinely sold and traded—in anonymized form—to third parties in for-profit commerce unrelated to their specific treatment. After a person gets medical care, pharmacies, insurers, labs, electronic record systems, and the middlemen connecting all these entities automatically transmit patient data directly to what is, in effect, a big health data bazaar. This trade—which has nothing to do with the individual’s treatment or insurance processing—is allowed by HIPAA privacy rules only if the patient’s name is removed. The result is a blizzard of transactions hidden to the public in which companies—called data miners—buy, sell, and barter anonymized but intimate profiles of hundreds of millions of Americans.
Such secondary use of patient data can have good intentions. For example, massive anonymized patient databases can help pharmaceutical companies develop and market effective drugs and treatments. The profiles that data miners produce remove the easy identifiers about a patient, such as name, birthdate, and so on, but they also leave certain information in the profiles, such as the doctor’s name, to allow drug companies to target sales to individual doctors based on their prescribing patterns.
While the anonymization of patient data may seem like a good firewall for protecting privacy, it increasingly is not. Because of the way big data can now be massaged and processed, companies can, for example, use the very same information to identify patients who are likely to suffer from certain conditions and then market drugs to them. Data scientists can now circumvent HIPAA’s privacy protections by making very sophisticated guesses, marrying anonymized patient dossiers with named consumer profiles available elsewhere—with a surprising degree of accuracy.
Beyond this well of anonymized data flowing from medical practices, there is also a flood of new information entering the data bazaar through nonmedical sources—none of which are protected by HIPAA. Social media, fitness devices, and health apps give advertisers additional information that can be openly traded and sold—but without any obligation to remove patient names or details. Online retailers selling health care products, such as books on back pain, or arm braces, can sell user profiles listing these items. It is no accident that a person with, say, carpal tunnel syndrome may see more Internet ads for products that match their specific medical condition: marketers may either know, or infer, someone is a receptive audience for their pitch.
This report unravels some of the secrets behind these complicated uses of medical big data, and argues that this impressive data wizardry actually poses significant risks. With lax rules, a massive trade in medical data has emerged that could result in serious negative consequences. Enough anonymized data gathered over time will eventually contain enough clues to re-identify nearly anyone who has received medical care, posing a big potential threat to privacy. Patient faith in the confidentiality of personal health information is essential to the effective functioning of health care, and that faith is likely to erode once the public learns about the extent of the current trade in their data.
Furthermore, an upsurge in hacking and medical data breaches is a parallel threat that can have potentially devastating impact. Unlike other hacks, such as credit card theft, the embarrassment and damage of a medical privacy breach cannot be undone: once intimate secrets are spread on the Internet, they do not disappear.
Because of these risks, policymakers need to strengthen health privacy rules and empower patients, rather than commercial companies, to determine what happens to their information.
U.S. Rules Governing Medical Data: What HIPAA Actually Says
Even medical professionals find the U.S. health privacy rules under HIPAA bewilderingly complicated. “HIPAA is so complex that I don’t know anyone who understands it,” says Warner Slack, a Harvard Medical School professor and one of the pioneers in developing electronic health records.1
Patients rarely comprehend how a medical entity uses and shares information, but sign HIPAA acknowledgement forms anyway (few realize they have the right to decline to sign, and still get treatment, according to U.S. Department of Health and Human Services guidelines).
HIPAA has limited reach, as it applies only to what are called covered entities. These include places of service and support that you typically would identify with medical treatment:2
- health care providers, such as doctors, clinics, nursing homes, and pharmacies;
- organizations covering the cost of health care, such as insurance companies, HMOs, company-funded plans, Medicare, Medicaid, the Veteran’s Health Administration, and other government medical programs; and
- health care clearinghouses, including any middlemen connecting the above health-related entities.
While HIPAA protects privacy in these facilities, the rules still allow these entities to sell or share patient data with outsiders unrelated to care, including for profit, if a patient’s name and certain identifiers are removed from each record. For example, a doctor or a lab performing tests on a cancer patient can sell the findings to a commercial company, provided they remove eighteen types of identifiers or have a statistician determine that there is a “very small risk” that the person could be re-identified.3 Few patients have any idea about this exception, which has allowed a multi-billion-dollar trade to evolve.
Furthermore, HIPAA rules do not apply to many types of health information, including:
- consumer medical-related purchases from non HIPAA-covered companies;
- health-related websites;
- Internet search engines;
- health clubs;
- fitness devices and health apps;
- marketing surveys and sweepstakes;
- GPS and mobile devices;
- life insurance and long-term insurance plans; and
- health inferences from lifestyle information collected by data brokers.
In sum, outside commercial companies can freely trade patient data anonymized to HIPAA standards, or identified information, including a person’s name, from areas outside HIPAA protection. Companies do not need to obtain a patient’s explicit consent before trading either type of information. “All evidence suggests the HIPAA standards are gravely inadequate,” writes Harvard Professor Latanya Sweeney, a top expert on medical privacy.4
The Commercial Trade in Anonymized Patient Data
Doctor-patient confidentiality dates back to the Hippocratic oath in ancient Greece. Yet the digitization of health-related records in recent decades has shattered this simple model. Today, companies known to few outsiders have built a multi-billion-dollar secondary market in anonymized patient information from billing, insurance claims, prescriptions, physician records, and other information related to medical treatment. Throughout the evolution of this trade, these firms have revealed little to patients about what they do. Doctors and others whose data is captured in this process have also often remained in the dark. Mapping this data network shows that the spread of patient data is surprisingly extensive (see Figure 1).
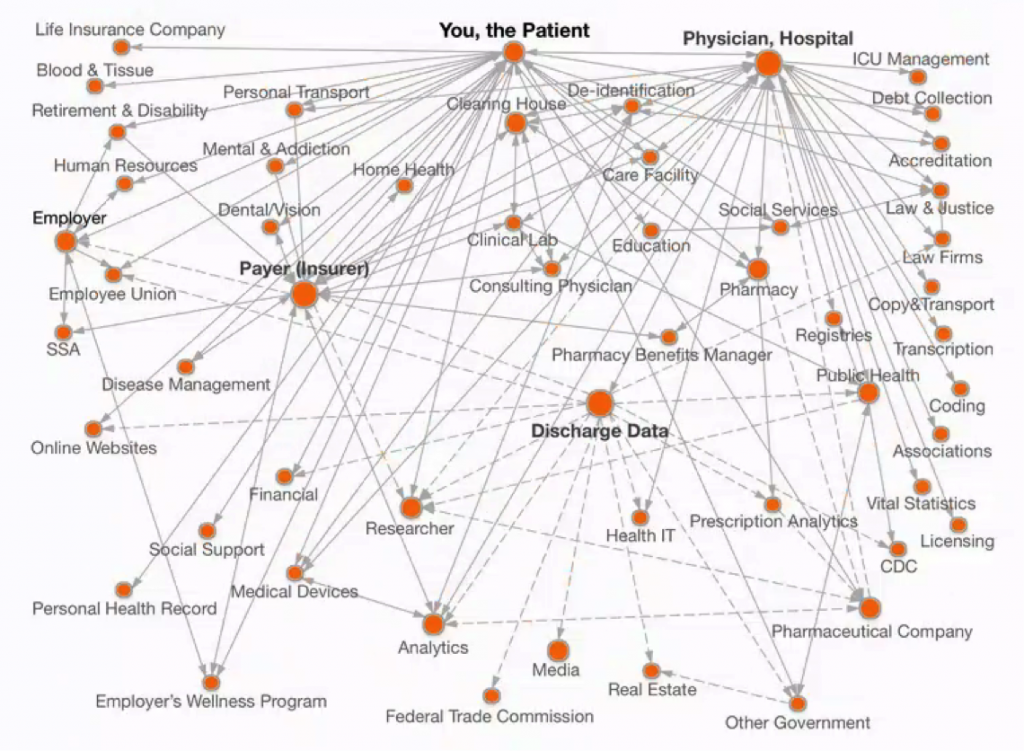
The Evolution of the Commercial Market in Patient Data
The origins of this secondary market date back half a century, with government inadvertently helping to plant its seeds by establishing Medicare and Medicaid in 1965. The need to process millions of medical-related records spurred computerization, which in turn created a steady supply of big data that outsiders could study.
By the 1970s, academics began analyzing anonymized claims records on hundreds of thousands of Medicare patients to identify trends and risks. These data pioneers then started working with large companies, such as General Motors and Johnson & Johnson, to better understand risks and costs among employees; several commercial data analytics companies emerged in the early 1980s.5 Some, such as MedStat Systems, offered companies free data analysis in exchange for the right to sell the information to drug companies, data mining companies, and others. The trade proved lucrative, and in 1994, MedStat’s founder sold the company to Canadian publisher Thomson Corporation for $339 million.6
Also in the early 1970s, pharmacies began to digitize their prescription records. The purpose was to improve health outcomes: such computerization allowed doctors to curtail adverse reactions by knowing what other drugs a patient takes, and to make sure multiple prescriptions are not given for the same condition. Over time, researchers began studying various databases to detect adverse drug reactions, including, for example, dangerous side effects from the drug Vioxx.7
The proliferation of digitized medical information created new commercial opportunities as well for health data mining companies, which started buying copies of pharmacy scripts to create doctor-identified reports that detail what medications individual physicians prescribe. Armed with such insights, pharmaceutical salespeople are able to tailor sales pitches carefully. Following HIPAA rules, such reports can include the prescribing doctor’s name—but not that of the patient—as well as details about the medication and dosage.
As this business first emerged, many pharmacies welcomed the chance to make extra money by selling information they were already gathering. “I got $50 a pop for that. And I thought I was making out like a bandit!” says Thomas Menighan, who opened a Medicine Shoppe franchise in Huntington, West Virginia in 1978.8
The dominant data mining company buying prescriptions has long been IMS Health (now called QuintilesIMS after a merger finalized in October 2016). Created in the late 1950s in a secret alliance between two major players in Madison Avenue medical advertising,9 IMS today obtains data on most prescription sales in the United States and in many countries abroad. After its recent merger, the stock market values the company at about $20 billion.
Pharmacies prefer not to announce that they sell their prescription information (even drug store employees are often unaware of the trade). “The patient is not really a component of this because their name and connection to the prescription have been stripped off,” says Per Lofberg, executive vice president of CVS Health. “Pretty much everyone who is in the business has some sort of supply arrangement for de-identified prescription data.”10
“Pretty much everyone who is in the business has some sort of supply arrangement for de-identified prescription data.”
For many years, doctors also did not know that outside firms were tracking their prescription habits. By the mid-2000s, enough had complained that several states in New England passed laws to ban the trade in doctor-identified data. IMS Health and other data miners sued; the Supreme Court overturned the laws on the grounds they restricted free speech.11
The Arrival of Longitudinal Patient Data
In the 1990s, data miners began aggregating files on individual patients, assembling dossiers about health conditions and medications taken over time, including how long someone stayed on a drug and what other medications he or she took. Such reports are anonymized in that they omit names and other easy identifiers, but they do include age, partial ZIP code, and other details.
Roger Korman, former president of IMS Canada and IMS Latin America during the period in which the company expanded its collection of individual data, explains how they approach pharmacies, doctors, insurers, and others when trying to buy their patient data. “We used to say, ‘Look, you are creating data as a byproduct. It’s an exhaust from your system. Why don’t you take that thing and turn it into an asset and sell it?’” he says. “That is the way we would get people to think about data as an asset—with full confidence that we were not violating anyone’s privacy or the law.”12
The growth of electronic health records and middlemen businesses connecting doctor offices with pharmacies and insurers has greatly eased the flow of such information sold to data miners.13 Health insurance companies have gotten into the longitudinal patient data business via separate units such as Optum (UnitedHealth) and HealthCore (Anthem). Blue Cross Blue Shield’s Blue Health Intelligence has data on 165 million people dating back to 2005,14 and supplies QuintilesIMS among others.
Companies best known for their work in other fields also aggregate massive patient databases. IBM Watson Health has expanded dramatically since its inception in 2015, including by purchasing Truven in 2016, an acquisition that gave them access to records on another 215 million patients. GE Healthcare shares details from anonymized data on 17 million patients in its electronic medical record system, Centricity.15 LexisNexis advertises it has gathered details on about 60 percent of all medical claims, with data from more than sixty health plans.
Overall, the improvement in computing speed and cheap storage has led to a dramatic growth in the scope and detail of individual anonymized patient files at the heart of this growing industry (see Figure 2). For example, data miner Symphony Health advertises that its Integrated Dataverse service has collected information on more than a dozen years of what they call the “patient’s journey” in the health system, including doctor and hospital claims and 90 percent of all U.S. prescriptions. QuintilesIMS has comprehensive dossiers on more than half a billion people worldwide.16
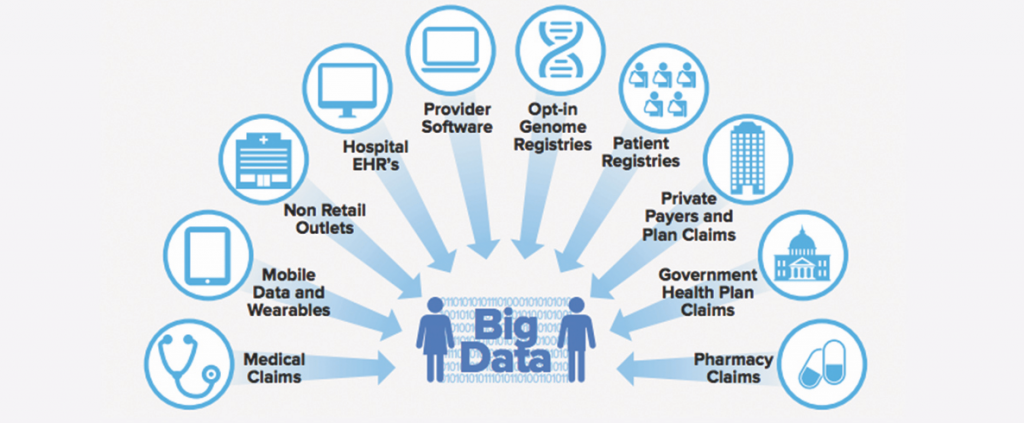
Data Brokers and Medical Information
In parallel to the commercial trade in anonymized medical data by data miners, related but different firms known as data brokers assemble and sell access to named files on most American consumers. Such identified information is exempt from HIPAA rules because it is gathered outside of health care providers and health plans and their intermediaries. Data brokers gather their health-related information from public records, surveys, loyalty programs, social media, commercial data such as magazine subscription lists, and other sources.
Information about someone from many different sources could suggest broader health insights. For example, algorithms might conclude that a deluxe cable TV package, alongside certain magazine subscriptions and clothing purchases, suggests a less healthy lifestyle. The data broker might then group together millions of people with similar attributes. “If people are filling out all these free coupons for free drugs and then you basically outline that information from some wholesalers that, hey, we have got 500,000 people who got a free booklet on arthritis or diabetics or whatever,” says Vin Gupta, a data broker entrepreneur. From that, one can infer “that these guys may have diabetics and they may have allergies.”17
Jennifer Barrett Glasgow, the chief privacy officer emeritus at leading data broker Acxiom, says they do not definitively know if someone suffers from a particular disease. “Health data that is sold by data brokers either comes from the consumer as self-reported on a survey or is inferred from retail, non-prescription purchases,” she writes. “In no way should this data be interpreted to mean the individual has the ailment, but instead it only indicates they have an interest in a medical condition.”18
Despite such cautions, some data brokers sell lists of people segmented by medical condition, often with address, phone number, or e-mail address. One website aggregating offerings from different data brokers includes the following lists:
- People With Cancer By State
- Booming Boomers With Erectile Dysfunction
- Bladder Control Product Buyers list
- Heart Disease Sufferers Email/Postal/Phone Mailing List
- STD Mater (of “mature singles that may have a sexually transmitted disease”)
Other brokers do not list specific medical ailments, but put people into marketing lifestyle categories. For example, Experian advertises a segment called “Kids and Cabernet,” in which people are “3.7 times more likely to say ‘I don’t take care of myself as well as I should.’” Epsilon, which has information on 250 million consumers, offers lists that include those interested in “Cigars and Tobacco.”19 Through splicing and dicing data, marketers could obtain very targeted lists of subpopulations divided into specific groups, such as, say, black men in California thought to have heart disease.
Consumers feed their information to these data brokers unwittingly because they do not understand the implications of health-related information shared outside a doctor’s office. “When companies in the data collection and processing business (like Google and Apple) expand to offer products in the health and wellness space, the line between patient and consumer is blurred,” says Michelle De Mooy, director for the Privacy & Data Project at the Center for Democracy & Technology. “Your understanding of the application of your health information in a medical context is fairly clear—your understanding of how it is used in a commercial context is far less clear and opaque.”20
New everyday devices equipped with web-connected sensors could have a profound but invisible impact. Sometimes called the “Internet of Things,” such items include devices as varied as smart thermostats, monitors beaming up data from automobiles, geolocators, cameras peering into refrigerators, even pillows and sex toys.
“Any device’s data may be used in far-removed contexts to make decisions about insurance, employment, credit, housing, or other sensitive economic issues,” writes University of Colorado School of Law Professor Scott Peppet. “Such data may lead to new forms of economic discrimination as lenders, employers, insurers, and other economic actors use Internet of Things data to sort and treat differently unwary consumers.”21
Medical Direct Marketing
In recent years, data scientists have also begun to combine dossiers about hundreds of millions of people from the separate realms of named data broker files and anonymized data mining dossiers. This sophisticated data alchemy, known as “propensity modeling,” does not break the de-identification of HIPAA-protected data. Rather, it uses that information to understand what types of people are most likely to have certain medical conditions based on their consumer profiles.
Once such a model is devised, health and medical-related companies can directly market from traditional data broker lists to named individuals who likely have an underlying health condition. “You end up with a much bigger, if you will, target audience,” explains Jennifer Barrett Glasgow, the Acxiom privacy official. “You have to understand that many people in the audience do not have the condition, but a lot of them, far more than in a normal audience, do have the condition. It does improve the marketing results without getting very, very specific.”22
One company that specializes in such modeling, Crossix, lists the following characteristics of those likely to suffer from hypertension: aged 55–70 professionals with graduate degrees and high incomes who are interested in art, travel, and home furnishings, among other attributes. “Our Consumer Health Portrait solution leverages past Rx and OTC usage information combined with consumer data to create deep profiles of your target audience,” the company says, referring to prescription and over-the-counter medication sales. “You can use these insights to improve audience segmentation and inform marketing planning & strategy.”23
Drug and medical companies can also draw more insights about consumers by monitoring visits to their web pages and cross referencing the data with data broker profiles on individuals. “Companies are really trying to not get directly identifiable data and not go directly back to the individual, but they are trying to get better at who they are profiling, about how the profiles look,” says lawyer Stan Crosley, a former chief privacy officer at drug maker Eli Lilly.24
Such techniques have clear advantages for marketers, but downside risks for consumers. Data algorithms hidden from the public could, for example, prompt life insurers to reject people based on their risk profile, or lead to redlining in which some people get worse insurance offers than others.25 “Is it appropriate to target a subpopulation you know is going to stay on the therapy while not targeting or reaching individuals who could still benefit from the therapy if they had either the finances or the knowledge about it?” Crosley asks.26
Because few consumers understand how data brokers and data miners operate—and these firms are often loath to detail their operations to regular people—all of this takes place behind the scenes in a system unknown to the public.
Anonymization and Re-Identification of Medical Data
The ability to gather anonymized data about a particular patient from different sources, or match identified and anonymized information in propensity modeling, has become more commonplace since the late 2000s, following advances in computing power and storage.
On the surface, it might seem impossible for a data miner to link anonymized information about a patient from separate sources—CVS at home in Cleveland today, but at Walgreens while on vacation in Miami Beach next month—or from different doctors in these cities. Yet data miners are able to match these files by getting pharmacies, insurers, testing labs, electronic health record systems, and other suppliers to all install the same de-identification software (for which they compensate the data suppliers).
This software removes the personal details for each individual—such as name, address, telephone number, and Social Security number—but assigns that person the same anonymous patient identification key across all locations using that de-identification system. “If they install that de-ID engine at every source and it has the same algorithm, that means everyone with the same PHI (personal health information) will get the same IMS patient key,” says Mark Degatano, who has advised IMS Health and worked at rival data miner Symphony Health.27
The “De-ID engine” allows data miners to assemble a patient dossier with thousands of data points spanning back years. The file does not include a name, but lists age and gender, as well as what section of Cleveland she lives in. Her doctors, whose information is not protected by HIPAA, can be listed by name.
The Growing Threat of Re-Identification
The same computing and storage power that facilitates de-identification makes it increasingly possible—and indeed likely, some experts reckon—that outsiders will be able to re-identify such files in the future. That is because so much data collection over time creates clues, like a fingerprint, that narrow down whose secrets might be held in the dossier.
Academic and journalistic experiments over the past two decades have illustrated how such re-identification works. In one well-known example, Latanya Sweeney, as a graduate student, showed that she could identify the overwhelming majority of anonymized patients whose insurance records the state of Massachusetts planned to release. She calculated that birth date, ZIP code, and gender alone offer enough clues to re-identify as many as 87.1 percent of all Americans. She later created aboutmyinfo.org that shows whether anyone else in the United States shares someone’s gender, birthdate, and ZIP code.
One researcher was even able to pinpoint celebrities among a database of New York City taxi passengers using only GPS data and ride time released by the New York City Taxi & Limousine Commission.
In other experiments, Sweeney re-identified volunteers who share their medical information with the Personal Genome Project, and used media articles to figure out the name of patients from anonymized records released by Washington State. Others have pinpointed individuals from among a list of anonymized Netflix users and from Internet searches made public by AOL. One researcher was even able to pinpoint celebrities among a database of New York City taxi passengers using only GPS data and ride time released by the New York City Taxi & Limousine Commission.28
“A lot of traditional thinking about anonymous data relied on the fact that you can hide in a crowd that’s too big to search through,” writes Arvind Narayanan, who re-identified Netflix users by matching them with named reviews on an online movie review site. “That notion completely breaks down given today’s computing power: as long as the bad guy has enough information about his target, he can simply examine every possible entry in the database and select the best match.”29
The information in these experiments was not anonymized to HIPAA standards. Nonetheless, ever-more granular details in anonymized patient dossiers, combined with the inherently identifying nature of DNA, will make assuring anonymity ever more difficult.30
“Bio-repositories that link genomic data to health care data are on the leading edge of confronting important questions about personal privacy in the context of health research and treatment,” a 2014 White House report noted.31
Ever More Medical Security Breaches
As medical practitioners have moved from paper to digital records, hacking and security breaches have become ever more commonplace.32 Rarely do more than a few days pass before the Department of Health and Human Services Office of Civil Rights posts details about the latest medical data breach. Some incidents involve a few thousand people; the worst case to date, involving insurer Anthem in 2015, impacted nearly 79 million patients (see Table 1).
Criminals have used such information for extortion, medical identity theft and fraud—such as to obtain free medical care or drugs—and to try to obtain tax refunds. Such information may be sold in shadowy online markets on the Deep Web, an area unknown to typical Internet users but easy for hackers to navigate.
“Cyber predators easily discover vulnerable systems in every healthcare organization like blaring beacons on a radar screen,” concluded a recent report by the Institute of Critical Infrastructure Technology. “The industry-wide refusal of executives to cyber hygienically evolve and expedite meaningful layers of cybersecurity can be directly correlated to the health sector remaining the most vulnerable to exploitation as well as the choicest of targets for adversaries seeking to maximize the financial payoff for their efforts.”33
Table 1. Recent U.S. Medical Security Breaches Involving More Than 20 Million People per Incident |
||
Company Suffering Data Loss | Total patients breached | Date of incident |
Anthem | 78,800,000 | 3/13/2015 |
Premera Blue Cross | 11,000,000 | 3/17/2015 |
Excellus Health Plan, Inc. | 10,000,000 | 9/9/2015 |
Science Applications International Corporation | 4,900,000 | 11/4/2011 |
University of California, Los Angeles Health | 4,500,000 | 7/17/2015 |
Community Health Systems Professional Services Corp. | 4,500,000 | 8/20/2014 |
Advocate Health and Hospitals Corporation | 4,029,530 | 8/23/2013 |
Medical Informatics Engineering | 3,900,000 | 7/23/2015 |
Banner Health | 3,620,000 | 8/3/2016 |
Newkirk Products, Inc. | 3,466,120 | 8/9/2016 |
21st Century Oncology | 2,213,597 | 3/4/2016 |
Xerox State Healthcare, LLC | 2,000,000 | 9/10/2014 |
IBM | 1,900,000 | 4/14/2011 |
Source: U.S. Department of Health & Human Services, Office for Civil Rights. |
The circulation of stolen medical information may also give outsiders clues to re-identify anonymized medical data. Purloined information could serve as an answer key to solve the de-identification puzzle, since information in stolen medical data and anonymized patient dossiers may detail the same treatment by a named doctor with certain procedure codes, times of treatment, and prescribed drugs.
Risk Scenarios
Secrets about health possess unique power to damage reputations, lead to discrimination, exclude someone from jobs and services, or wreak other damage. Actor Charlie Sheen knows about this negative impact all too well. In 2015, he admitted that he had paid millions of dollars in blackmail to hide his HIV positive status. “To date, I have paid out countless millions to these desperate charlatans,” Sheen wrote in an open letter. “Locked in a vacuum of fear, I chose to allow their threats and skullduggery (sic) to vastly deplete future assets from my children, while my ‘secret’ sat entombed in their hives of folly (or so I thought).”34
People far from the glamour of Hollywood also face real risks.
People far from the glamour of Hollywood also face real risks. A rival may gather enough clues about a co-worker’s medical history to re-identify anonymized records. A nosy neighbor, a romantic rival, or business competitor—many people could benefit from revealing sensitive medical information.
This dark side of the big health data bazaar could result just from unleashing sensitive details about someone’s body or mind on the Internet. Similar practices already occur when hackers publish home and e-mail addresses, Social Security numbers, and financial information of celebrities, politicians and others in a practice called “doxing.” Revenge porn—the publication of intimate images or videos without the consent of the filmed person—is another variant of public humiliation.35 A data hunter might also release medical information selectively, by sending medical details about someone to a boss, spouse, priest, or journalist.
Medical data used as a weapon could also take on political or diplomatic dimensions. The bitterness of U.S. politics in recent years makes it easy to imagine murky political operatives re-assembling patient records about an opponent. With foreign government hacking against U.S. targets on the rise, experts in Russia, China, or North Korea could seek to identify medical records to humiliate or even blackmail public officials.
Such attacks could target U.S. military personnel, seeking to cause damage on the home front far from the battlefield. Even anonymized medical information could give an adversary military insights into health trends in a select area such as around a military base. For example, an upsurge in anthrax vaccinations around Fort Bragg, North Carolina, might reveal clues into future U.S. special forces military intentions.
The Risk to Patient Confidence in the Medical System
The commercialization of what takes place in the doctor’s office, hospital room, or blood lab has happened without public debate, with many doctors and pharmacist themselves unaware that their work generates a commercial product. This unfettered trade threatens to erode public trust in health care practitioners—once people realize their medical secrets are in circulation. Patients may prove more reluctant to detail intimate problems if they know that such information forms part of a dossier in cyberspace unrelated to their care and whose ultimate use is completely outside of their control.
The Limited Impact of Big Data on Medical Research
Data miners and companies trading patient information argue that privacy threats from the wide circulation of anonymized data are exaggerated. They counter that what they see as small risks are worth taking because of the promise of new discoveries and insights from the wide commercial circulation of anonymized patient information. “The future of medicine rests on data: the evidence that is the basis for the discovery, development and dispensing of prescription products and all other healthcare decisions,” QuintilesIMS wrote in an October report that highlights the importance of data in medicine. “Mastering the collection and interpretation of data is therefore vital for the vitality and continued global contributions of the biopharmaceutical industry.”36
In reality, anonymized medical data that is commercially circulated without patient consent has, so far, fallen short of delivering any major medical breakthroughs. Throughout the history of commercial data mining, sales and marketing rather than science has propelled the trade. “It’s much more hype than promise at this point, hype than reality,” says Caleb Stowell, a vice president of standardization at the International Consortium for Health Outcomes Measurement, a nonprofit group founded by Harvard’s Institute for Strategy and Competitiveness, the Boston Consulting Group, and the Karolinska Institutet in Sweden. “Much of the data they are collecting we don’t think have much value.”37
Asked to cite the most significant breakthroughs from the study of anonymized patient information, data miners speak of cost efficiencies or interesting insights rather than cures to diseases or dramatic breakthroughs.38 “The reality is that data-driven benefits for health care have still not materially showed up,” says Kris Joshi, executive vice president at Change Healthcare (formerly Emdeon). “Health care is generating a very, very pitifully small amount of value given the amount of data there.”39
Part of the problem in advancing science through commercial data mining is that such files are an aggregation of what firms are able to obtain commercially, not that which scientists would set out to obtain in ideal research conditions. Some testing labs sell their data to data brokers, others do not, as is the case with insurers and others gathering patient data.
The gold standard in medical research remains randomized clinical trials, in which different groups of people randomly receive different treatments so that researchers—who do not know which patients get which medications—can test which proves most effective.
“You need large scale randomized evidence to answer a lot of questions, and I think the claim that database analysis will do so isn’t justified,” says Richard Peto, an Oxford professor of medical statistics and epidemiology who has long studied the causes of cancer and the impact of smoking. “I am not saying that nothing is going to come out of analyzing lots and lots of medical records. But I think what is claimed is that you can often make lots of conclusions about which treatments work and which don’t and I think that is not true, that you can’t sort that out reliably from medical records and who got which treatment.”40
Policy Recommendations
Given the growing risks of trafficking in anonymized medical information, past rules have become increasingly inadequate in protecting patient privacy. Thus broader protections than HIPAA now offers are needed. However, a change in government rules that empowers patients with greater control need not curtail future scientific research into medical big data.
Extend Privacy Protections to Anonymized Data
HIPAA’s limited scope, combined with rapid progress in storing and processing digital information, has allowed a big health data bazaar to evolve that few outside of health care know about, that data miners are reluctant to discuss, and that has the potential to pose real harm to patients.
Legal protections should extend to “an individual’s health information,” not just to the category of “individually identifiable health information” covered by current regulations.
Any and all individuals should have a say in any secondary use of their medical data. Legal protections should extend to “an individual’s health information,” not just to the category of “individually identifiable health information” covered by current regulations. Such a change would bolster patient trust in the health care system by insuring the confidentiality of information provided to medical practitioners. Protecting health data more broadly would bar the sale of anonymized medical data without informed patient consent, and would apply to pharmacies, health information systems, insurers, labs and others buying and selling such information.
After HIPAA is changed to include anonymized data, a person should also be able to ask data miners such as QuintilesIMS and Symphony Healthy to remove any anonymized details about them already in circulation.
Broaden Protections to More Types of Health-Related Data
HIPAA-style protections should extend to medical-related data gathered by websites and forums, fitness apps, and the Internet of Things.
Because it is difficult to draw a line between medical and non-health related information gathered by data brokers and others, wider privacy protections may be in order. Lifestyle details can give outsiders significant insights into a person’s health: if an Internet-connected pan knows its owner is frying up bacon every day, that insight, combined with other pieces of information, could lead to health-related discrimination.
The Federal Trade Commission has suggested that rules pertaining to the fast-expanding Internet of Things be part of broader Congressional legislation to update privacy regulations.41 In expanding protections to a wider array of personal information, the European Union provides one model with its General Data Protection Regulation, which takes effect in 2018.42
Explain Sharing Choices in Plain English
To better inform the public about what happens to their data, policy makers should require entities handling personal information to use clarity and plain language in privacy statements. One possible model comes from financial institutions which send out standardized privacy policies with (relatively) comprehensible charts with categories including: “Reasons we can share your personal information,” “Does the bank share your data?” and “Can you limit this sharing?”43 New European Union rules provide possible guidelines to emulate: “The request for consent must be given in an intelligible and easily accessible form, with the purpose for data processing attached to that consent.”44
The bottom line is that before trading a person’s data commercially—even if anonymized—marketers and others must obtain clear, knowing consent—not a click on an Internet page following five thousand words of impenetrable “terms of use” prose whose meaning would challenge even a seasoned lawyer to understand.
Empower Patients to Decide on Sharing Data
Empowering patient control of intimate information need not curtail big health data research. Allowing patients to decide simply means that profit-seeking commercial companies outside the public eye will not be able to dictate quietly what is best for everyone.
Patients themselves should determine whether and with whom to share anonymized data to help research. One approach might be a centralized list, akin to the National Do Not Call Registry, that records a person’s consent to anonymized sharing to select entities. Such a registry would allow easy sharing without seeking the patient’s preference at every medical visit or transaction.
Surveys have shown that many people will donate to science if asked. A 2015 Truven-NPR poll of 3,010 U.S. adults found 53 percent willing to contribute health information anonymously, with younger people more willing than their elders. 45 A similar question a year prior found 68 percent ready to share.46 Other surveys suggest the numbers could be even higher if people understand the concrete benefits, such as identifying potential drug safety issues or reducing costs for treating diseases.47
How patients are empowered with choice will dramatically impact how many end up sharing, because most people will go with the default and not act in any way. For example, countries that automatically donate organs to medicine upon death unless a person opts out have far higher participation rather than those that ask for explicit permission by creating an opt-in system.48
The most patient-empowering approach would allow people to gather their own comprehensive records electronically, and then share them directly, via an easy Internet interface, with whatever entities or research projects they wish.49 “We don’t ask people for their preference on kinds of charity they approve of and then take money out of their bank without notice. We ask people to donate to a particular charity on a particular day. The same should be true for personal data,” says Adrian Gropper, chief technology officer of Patient Privacy Rights, an advocacy group.50
Others suggest that the best balance between “opt in” and “opt out” would be “forced choice” or “no default.” That means that patients would not automatically share data unless they opt out or have to opt in proactively to share data. Rather, they would face an explicit “share or not share” choice between two checkboxes of equal size. Such a choice option might also allow patients to share their information only with scientific researchers, or also include commercial companies, an option available to volunteers in the famous Framingham Heart Study.51
Support Noncommercial Research using Patient-Consented Data
This report focuses on use of data by commercial entities that profit from trafficking in anonymized patient data. Yet society can and should help academic researchers harness the power of big data for the future. For example, the government can facilitate sharing by making national databases accessible to qualified researchers. One recent U.S. government initiative, the Precision Medicine Initiative Cohort Program, is hoping that a million people will volunteer their DNA samples and medical histories for scientists to study.
In discussing the program, President Barack Obama expressed privacy concerns about such research. “We’ve got to figure out, how do we make sure that if I donate my data to this big pool, that it’s not going to be misused, that it’s not going to be commercialized in some way that I don’t know about,” he said. “And so we’ve got to set up a series of structures that make me confident that if I’m making that contribution to science that I’m not going to end up getting a bunch of spam targeting people who have a particular disease I may have.”52
Some nations consider medical data sharing part of the social compact, with Nordic countries a notable example. Sweden maintains national databases with heart disease, cancer, and HIV patients, and hopes they will help researchers improve the lifespan of patients and the cost effectiveness of treatment.53 U.S. government entities, including the Centers for Medicare & Medicaid Services, release some anonymized claims information without patient consent, as do many U.S. states with their “all-claim patient” and other databases consisting of medical, pharmacy and other medical claims.54
Dr. Chesley Richards, director of the Office of Public Health Scientific Services at the Centers for Disease Control and Prevention, suggests the best model for the United States might be a public-private health data partnership. “I don’t see it as an individual company or an individual business and government, I see it as a board of some type, some sort of framework like that that can set standards,” he says.55
One effort in this direction is the Health Care Cost Institute, a nonprofit U.S. research group which has collected anonymized information on more than 50 million patients from Aetna, Humana, and the UnitedHealth Group and limits its sharing to academic researchers.
Even if a government entity shares anonymized data, a patient should still have a say on participating, a choice that Rhode Island pioneered in 2014 by allowing residents to opt out of its anonymized state insurance claims sharing. A more patient-empowering approach would require patients to explicitly agree to sharing, with exceptions for important public health purposes such as tracking deadly epidemics.
Encourage Public Discussion of this Complicated Issue
Because issues surrounding patient data are complicated and nuanced, an open public dialogue is essential to forge the best public policy. Even a report of this length can only touch on some of the many complicated issues around medicine, big data, and privacy. For-profit businesses should not be allowed to follow their secretive instincts and establish by fiat the standards that impact hundreds of millions of Americans.
Because issues surrounding patient data are complicated and nuanced, an open public dialogue is essential to forge the best public policy.
To date, key players in the big health data bazaar have revealed few details about the working of their businesses. Very few patients are aware of the extensive trade in their data. Yet transparency is essential to provide stronger privacy protections and to encourage the responsible use of information to advance science. This dialogue should include patients, health care providers, researchers, policymakers, industry officials, journalists and others. The recommendations of this paper should be viewed as just a start to such a dialogue aimed at improving and evolving a better balance between patient privacy and the potential of big medical data.
Conclusion
Patients need to gain control over the fate of their medical information, whether identified or anonymized, and they should be able to determine whether or not to share such data for science or commerce. Companies handling this information must offer far more transparency. At stake is patient confidence in the entire health system. People must feel assured that what they tell health practitioners behind closed doors will stay private and not become a commercial product without their consent.
This report is supported in part by a grant from the Open Society Foundations.
Notes
- Interview with author, September 9, 2015.
- There are many exceptions among those entities one might expect to be subject to HIPAA, such as employer health plans impacting fewer than fifty employees. The Department of Health and Human Services (HHS) sets out some of the exceptions and broad guidelines in this “Summary of the HIPAA Privacy Rule,” archived at https://perma.cc/6E5L-YENQ.
- HHS explains the de-identification guidelines on “Guidance Regarding Methods for De-identification of Protected Health Information in Accordance with the Health Insurance Portability and Accountability Act (HIPAA) Privacy Rule,” March 2010, archived on https://perma.cc/932L-JDUB.
- Latanya Sweeney, letter to the Department of Health & Human Services, Office of the Secretary, October 26, 2011, archived at https://perma.cc/8NR8-78D2. Full disclosure: I have worked closely with Sweeney in recent years as a fellow at Harvard.
- Early data analytics companies studying claims information included Health Data Institute and MedStat Systems. For one early study of health care costs using such data, see Linda Demkovich, “Controlling Health Care Costs at General Motors,” Health Affairs 5, no. 3 (August 1986): 358–67, https://perma.cc/J47Q-T8PV.
- For details on the sale, see Lawrence Fisher, “Thomson Will Buy Medstat for $339 Million,” New York Times, November 17, 1994, http://www.nytimes.com/1994/11/17/business/company-news-thomson-will-buy-medstat-for-339-million.html.
- A 2002 study, using data from the Tennessee Medicaid program, identified a higher risk of heart disease for those using Vioxx. See Wayne Ray, Michael Stein, James Daugherty, Kathi Hall, Patrick Arbogast, and Marie Griffin, “COX-2 selective non-steroidal anti-inflammatory drugs and risk of serious coronary heart disease,” The Lancet 360, no. 9339 (2002 ): 1071–73. A later study confirmed those findings. D. H. Solomon, S. Schneeweiss, R. J. Glynn, Y. Kiyota, R. Levin, H. Mogun, and J. Avorn, “Relationship between selective cyclooxygenase-2 inhibitors and acute myocardial infarction in older adults,” Circulation 109, no. 17 (May 2004) :2068–73.
- Menighan, quoted in Our Bodies, Our Data: How Companies Make Billions Selling Our Medical Records (Boston: Beacon Press, 2017).
- The behind-the-scenes history of IMS and its evolution is told in Our Bodies, Our Data.
- June 9, 2014, interview with author.
- See Supreme Court decision, Sorrell v. IMS Health Inc., No. 10-779 131 S.Ct. 2653 (2011), https://perma.cc/9VSH-KY35.
- Interview with author, December 2, 2014.
- Sales can also bring in significant income for suppliers. For example, electronic records company Allscripts, which says their systems are used by half of all hospitals and in a third of U.S. doctor offices, makes $30 million a year in data sales. Allscripts revenue numbers from mid-2015 by Faisal Mushtaq, general manager of Allscript’s payer/life sciences business, quoted in Tanner, Our Bodies, Our Data. The growth has come as the Health Information Technology for Economic and Clinical Health (HITECH) Act passed under President Barack Obama has paid many billions of dollars of government subsidies to spur the widespread adoption of electronic health record systems in medical offices and hospitals.
- Statistic from BHI’s website, “Meet the Future Head On, Today,” 2016, archived at https://perma.cc/C5N8-V5FJ.
- For example, GE advertises an “easy-to-use, hosted service that leverages a secure Internet connection and HIPAA-compliant process to extract anonymous, de-identified patieint clinical data and send it to a centralized data warehouse every night.” See “Strength in Numbers,” GE Healthcare, 2010, at https://perma.cc/UFP3-38CZ. The 17 million patient number comes from “Centricity Electronic Medical Record,” GE Healthcare, 2010, archived at https://perma.cc/J2A5-6W4T.
- The number of longitudinal patient files comes from “Annual Report,” IMS Health, 2015, p. 4, archived at https://perma.cc/866F-TN6Y.
- Interview with author, November 8, 2012.
- Glasgow quoted in her posting “Responsible Use of Consumer Data: Fact or Fiction,” Acxiom, March 17, 2014, archived at https://perma.cc/2EFV-N9NJ.
- Adam Tanner, What Stays in Vegas: The World of Personal Data-Lifeblood of Big Business-and the End of Privacy as We Know It (New York: PublicAffairs, 2014), 79, http://www.publicaffairsbooks.com/book/hardcover/what-stays-in-vegas/9781610394185.
- E-mail to author, February 9, 2016.
- Scott R. Peppet, “Regulating the Internet of Things: First Steps Toward Managing Discrimination, Privacy, Security & Consent,” Texas Law Review 93, no. 1 (March 1, 2014).
- Interview with author, July 7, 2015.
- Details of the hypertension profile and quote come from Crossix webpage archived https://perma.cc/C2B5-H9QQ. The company has said that it works with some of the biggest names from both sides of the personal data world, such as data broker Acxiom and medical data miner Optum.
- Interview with author August 13, 2015.
- Mathematician Cathy O’Neil writes about how personal data entered into the black boxes of data algorithms can have a profound impact on someone’s life in her recent book Weapons of Math Destruction: How Big Data Increases Inequality and Threatens Democracy (New York: Crown Random House, 2016), https://weaponsofmathdestructionbook.com/.
- Ibid.
- Interview with author, June 8, 2015.
- The studies cited in this paragraph are from Latanya Sweeney, Akua Abu, and Julia Winn, “Identifying Participants in the Personal Genome Project by Name,” Data Privacy Lab, April 29, 2013; Latanya Sweeney, “Matching Known Patients to Health Records in Washington State Data,” Data Privacy Lab, arXiv:1307.1370, July 5, 2013; Arvind Narayanan and Vitaly Shmatikov, “Robust De-Anonymization of Large Sparse Datasets,” Proceedings of the 2008 IEEE Symposium on Security & Privacy (2008): 111–125; Michael Barbaro and Tom Zeller Jr., “A Face is Exposed for AOL Searcher No. 4417749,” New York Times, August 9, 2006; blog post, Anthony Tockar, “Riding with the Stars: Passenger Privacy in the NYC Taxicab Dataset,” Neustar Research, http://www.nytimes.com/2006/08/09/technology/09aol.html.
- From Arvind Narayanan’s website, “33 Bits of Entropy,” archived at https://perma.cc/4N7H-GP4S.
- At a time that direct-to-consumer DNA testing is growing in popularity, it is worth remembering that some testing companies share this revealing data by default, and others, such as 23andMe, encourage customers to agree to do so to help scientific research. For more details see Adam Tanner, “The Promise & Perils of Sharing DNA,” Undark, September 13, 2016, archived at https://perma.cc/4DQZ-G9HR.
- Executive Office of the President, “Big Data: Seizing Opportunities, Preserving Values,” Washington D.C., May 1, 2014.
- For more details see Adam Tanner, “Data Thieves Find Easy Pickings in the Health Care System,” Scientific American, July 27, 2016, archived at https://perma.cc/6TFP-Z4RN.
- James Scott and Drew Spaniel, “The Deep Web Exploitation of Health Sector Breach Victims,” Institute of Critical Infrastructure Technology, September 22, 2016.
- Charlie Sheen, “Open Letter on HIV-Positive Diagnosis,” NBC Today, November 17, 2015, archived at https://perma.cc/8W5Z-UBUH.
- For more on the issue of revenge porn and the business of humiliation, see Adam Tanner, “Legal Questions Raised by the Widespread Aggregation of Personal Data,” New England Law Review 49 (2015): 601, archived at https://perma.cc/P6ZP-G497.
- QuintilesIMS Institute, “Connecting Insights,” October 2016, summary archived at https://perma.cc/4UTR-A5NM.
- Interview with author, November 4, 2016.
- QuintilesIMS says their aggregation of patient information significantly helps science, and publishes a list of academic research based on their data: “Advancing Academic Research,” IMS Institute for Healthcare Informatics,” May 2016, http://www.imshealth.com/files/web/IMSH%20Institute/IMS_Institute_Bibliography_June_2015.pdf, archived at https://perma.cc/7F4R-7FB4.
- Interview with author August 21, 2015.
- Interview with author, October 13, 2015.
- FTC Staff Report, Internet of Things, Privacy and Security in a Connected World, January 2015, viii. Archived at https://perma.cc/2GD9-LCQR.
- A useful summary of the new EU rules comes from “The EU General Data Protection Regulation,” Allen & Overy, 2016, archived at https://perma.cc/EHP6-AW7M.
- In advising businesses about the requirements for financial data covered under the Gramm-Leach-Bliley Act, the Federal Trade Commission gave the following guidelines archived at https://perma.cc/CJB7-589B: “The notice should use plain language, be easy to read, and be distinctive in appearance. A notice on a website should be placed on a page that consumers use often, or it should be hyperlinked directly from a page where transactions are conducted.”
- “General Data Protection Regulation,” EUGDPR.org, archived at https://perma.cc/KLD4-BT8S.
- Truven Health Analytics–NPR Health Poll, January 2015, archived https://perma.cc/Q86J-ERY2.
- This British study further explores patient attitudes toward sharing data: K. Spencer, C. Sanders, E. A.Whitley, D. Lund, J. Kaye, W. G. Dixon, “Patient Perspectives on Sharing Anonymized Personal Health Data Using a Digital System for Dynamic Consent and Research Feedback: A Qualitative Study,” Journal of Medical Internet Research 18, no. 4 (2016): e66, archived at https://perma.cc/H5RK-2BB9.
- Truven Health Analytics–NPR Health Poll, November 2014, archived at https://perma.cc/ZSC9-7W6K.
- This article by Eric Johnson and Daniel Goldstein explores the impact of choice on organ donation and includes a chart showing the dramatic difference of opt in or opt out: Eric Johnson, and Daniel Goldstein, “Do Defaults Save Lives?” Science 302 (November 21, 2003): 1338–39, available at https://ssrn.com/abstract=1324774.
- To date, patient access to comprehensive records remains rare, despite billions of dollars in U.S. government subsidies to encourage the digitization of medical records. A relatively small percentage of total patients use services such as Microsoft HealthVault aimed at giving patients access and control of their records.
- E-mail to author November 11, 2016.
- The Framingham Heart Study provides links to its various consent forms: “Framington Heart Study,” National Heart, Lung, and Blood Institute and Boston University, archived at https://perma.cc/62HF-D4FH. One example is the following consent question: “|___| YES |___| NO I agree to allow researchers from private companies to have access to my DNA and genetic data which may be used to develop diagnostic lab tests or pharmaceutical therapies that could benefit many people.”
- White House transcript, “Remarks by the President in Precision Medicine Panel Discussion,” February 25, 2016, archived at https://perma.cc/7FH7-EF5D.
- This article reviews ethical questions related to Nordic registries. Some registries allow researchers access to identifiable data: Jonas F Ludvigsson, Siri E Håberg, Gun Peggy Knudsen, Pierre Lafolie, Helga Zoega, Catharina Sarkkola, Stephanie von Kraemer, Elisabete Weiderpass, Mette Nørgaard, “Ethical aspects of registry-based research in the Nordic countries,” Clinical Epidemiology 7 (November 23, 2015): 491–508.
- “Identifiable Data Files,” Centers for Medicare & Medicaid Services webpage, November 2016, archived at https://perma.cc/EX23-RUTN. Proponents of programs facilitating the sharing of anonymized patient information say citizens should feel obliged to share to help society. “While I approve that your data is yours, I also believe that the public health is more important than your personal ownership of health care data,” says Joel Kallich, a health economist and consultant whose past clients include IMS. “In other words, it is a trade off, you want to live in this society, get health care that is paid for by all of us, then you have to give up some of your rights to your data.”
“But the partnership that should exist between those whose data is being used, and those who are using it for various projects should be clear, as researchers owe people who provide their data for research, clear information on how, what and why their data is being used.” E-mail to author, November 17, 2016. - Interview with author.